Uses of noise in neuron-neuron communications
This is a hot topic, and in the past week two papers have brought new perspectives:
1) Cafaro and Reike (here) discuss how correlated noise between inhibitory and excitatory inputs are necessary for neurons to effectively integrate those signals. They show this by recording the activity of retinal neurons in response to light.
As a control, they find cross-correlations in signal activity (using MATLAB's xcov) when the two neurons are recorded simultaneously, but not non-simultaneously. Then, they estimate variability in synaptic responses by subtracting the average synaptic input from each individual trial. The peak correlation of these residuals ranges from 0.15 to 0.5 when the signals are recorded simultaneously. Noise correlations in non-simultaneous recordings were much smaller, but nonzero, which they attribute to a slow drift in the stimuli response.
Finally, when they eliminate this correlated noise, it decreases the accuracy of the neuron's spiking responses to the light input.
2) Schwalger et al (here) study the impact of noise on neural interspike interval stats. In particular, they distinguish between two different types of noise: 1) fast fluctuation noise, which comes mainly from ion channel noise, due largely to the speed of ion conductance at the synapse, or 2) slow adaptation, which could come from calcium fluctuations in calcium-gated potassium currents, like BK channels.
As you can see below, simulations show that these types of noise produce different of interspike interval histograms. In particular, the model which includes slow adaptation noise, linear and B and log-log in D, cannot be fit by the inverse gaussian distribution.
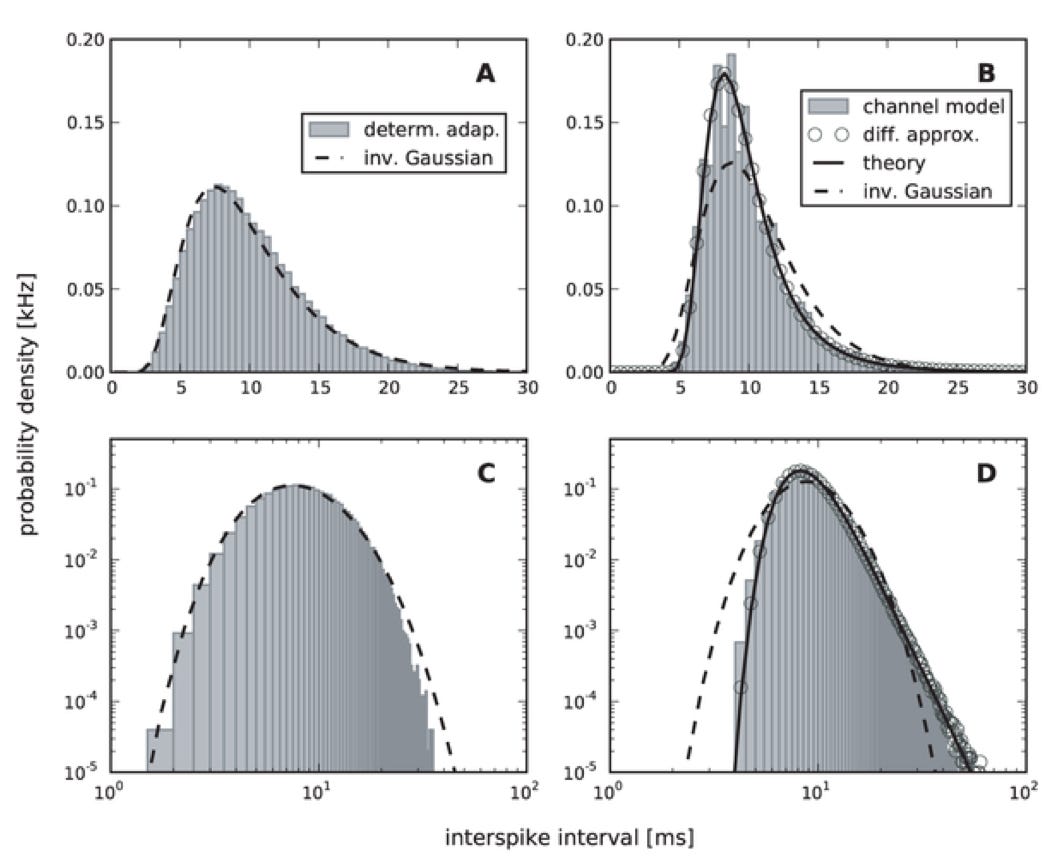
As you can see, simulations dominated by slow adaptation noise yielded distributions with higher skewness and kurtosis. The authors suggest that this might allow interspike interval recordings to delineate the main contributor to noise in a given neuron or class of neurons.
Noise is inevitable in biological systems, and this is especially the case in neurons. The first paper shows one way organisms are able to not only cope with noise but actually make use of it. The second paper suggests that some neurons, depending on the sources of their noise, might have qualitatively different rates of spiking.
References
Cafaro J et al. 2010 Noise correlations improve response fidelity and stimulus encoding. doi:10.1038/nature09570
Schwalger T, Fisch K, Benda J, Lindner B (2010) How Noisy Adaptation of Neurons Shapes Interspike Interval Histograms and Correlations. PLoS Comput Biol 6(12): e1001026. doi:10.1371/journal.pcbi.1001026